New 最新上架
Top videos 最受歡迎
TV Programs 電視節目
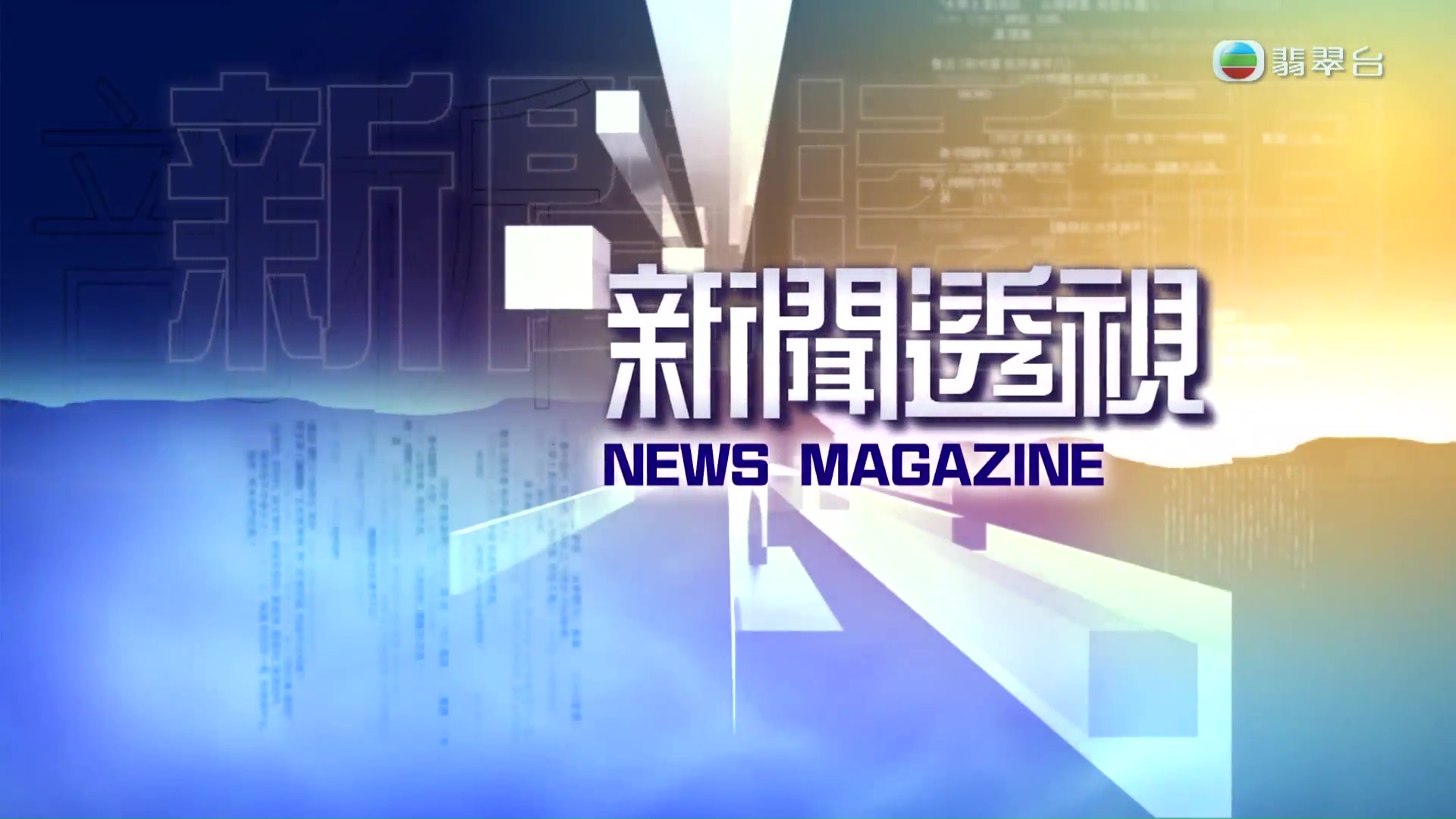
新聞透視
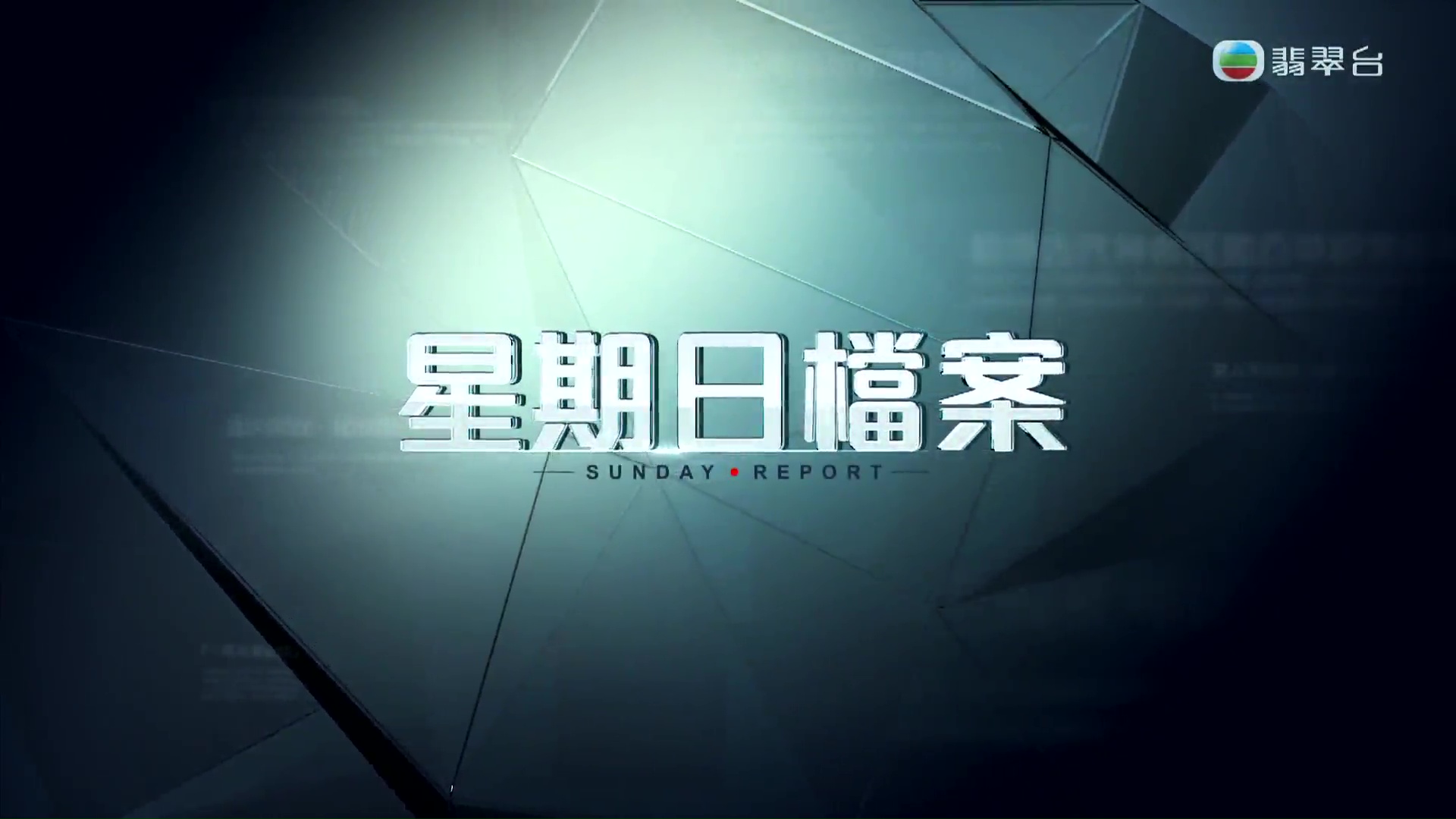
星期日檔案
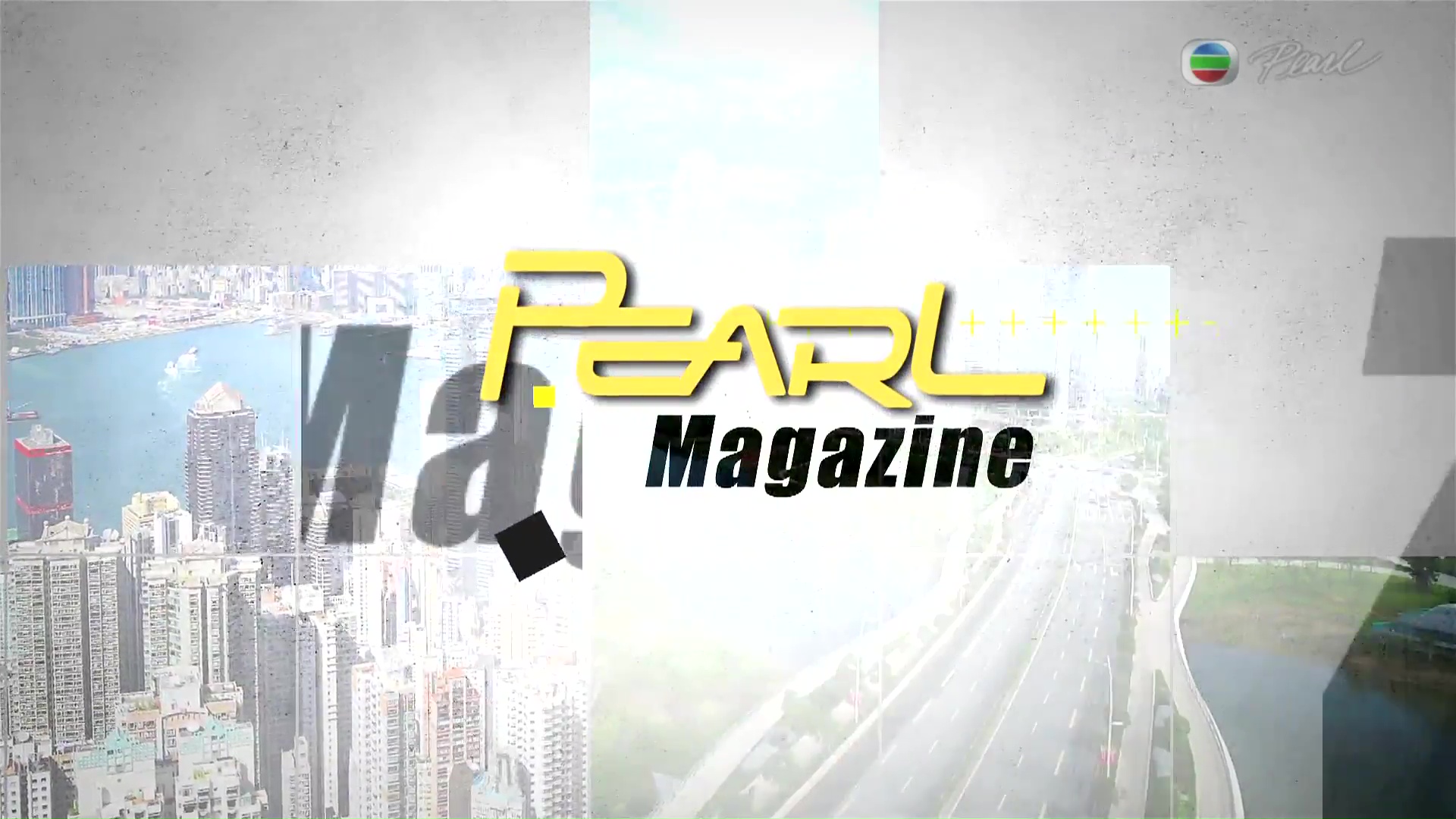
Pearl Magazine
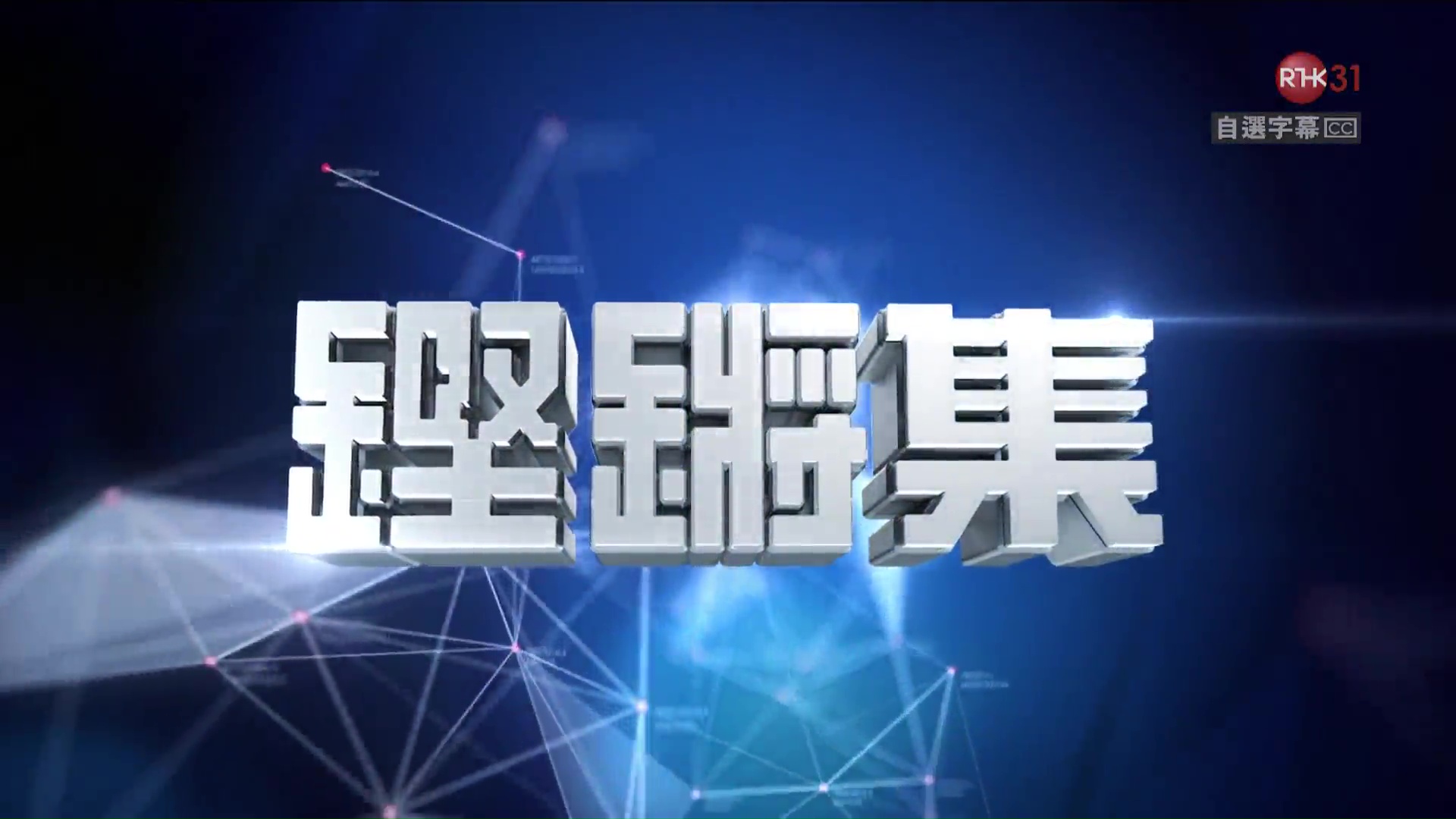
鏗鏘集
Scholarly Talks 學術論壇
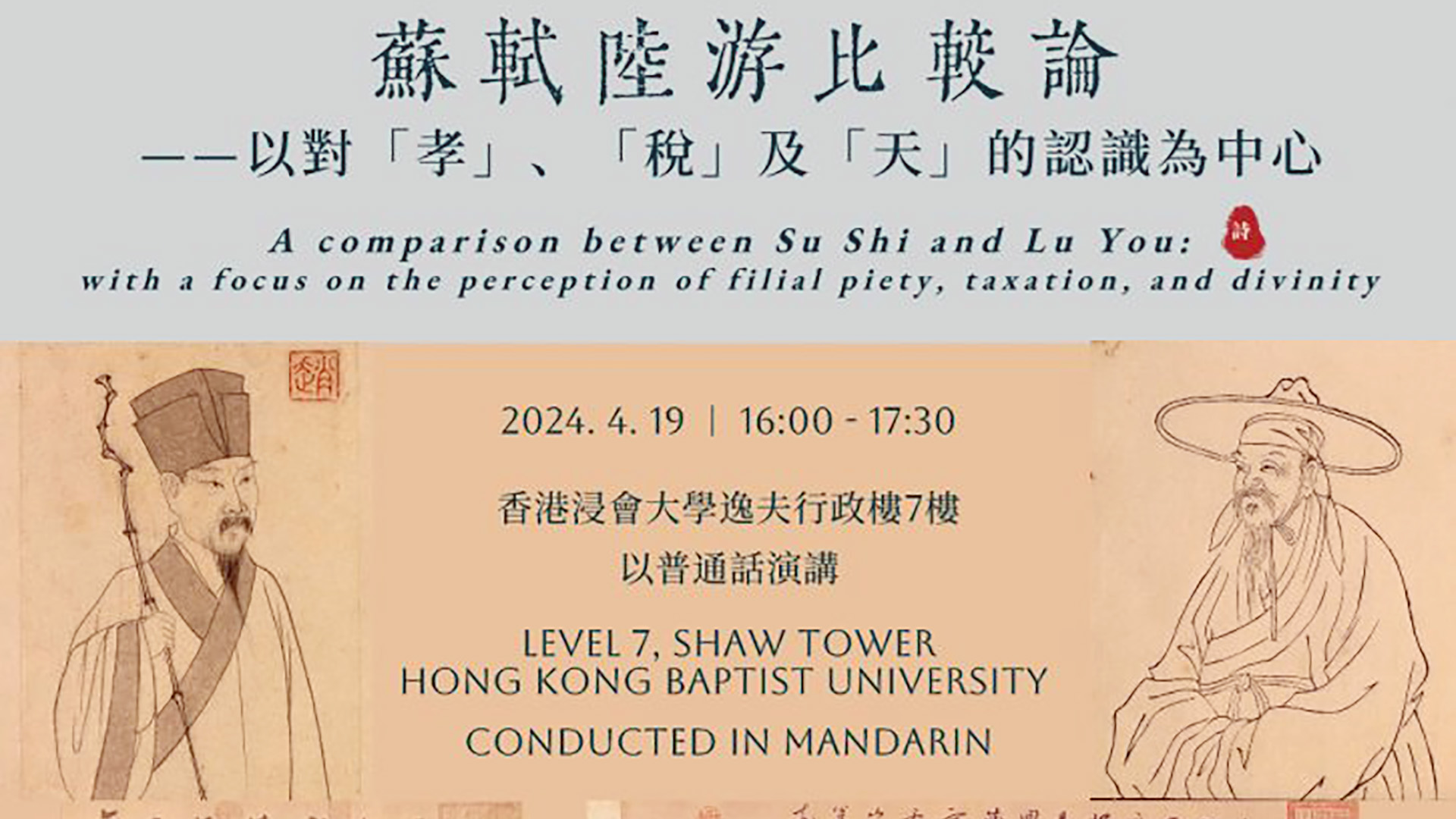
苏轼陆游比较论——以对「孝」、「税」及「天」的认识为中心
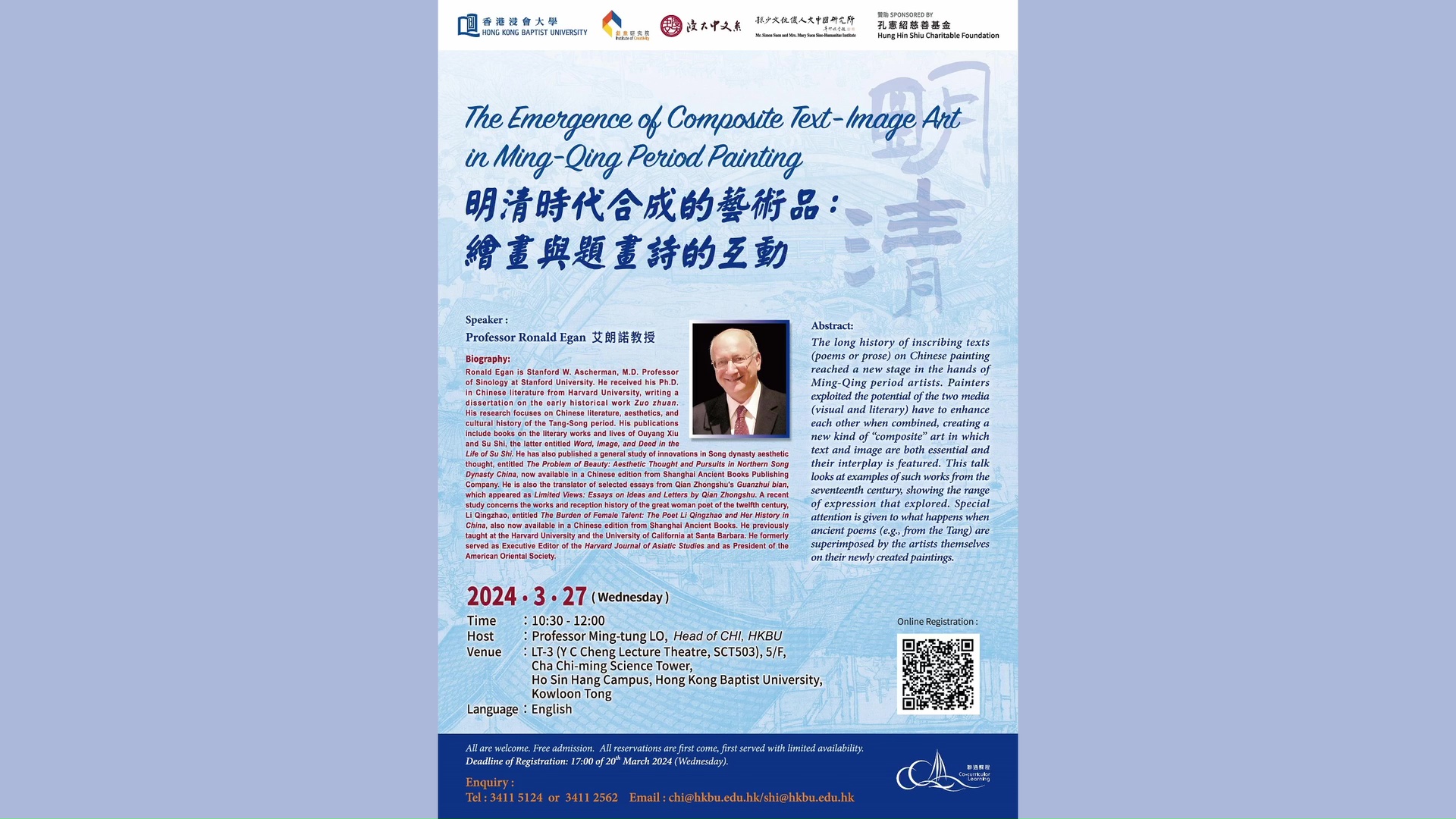
The Emergence of Composite Text-Image Art in Ming-Qing Period Painting
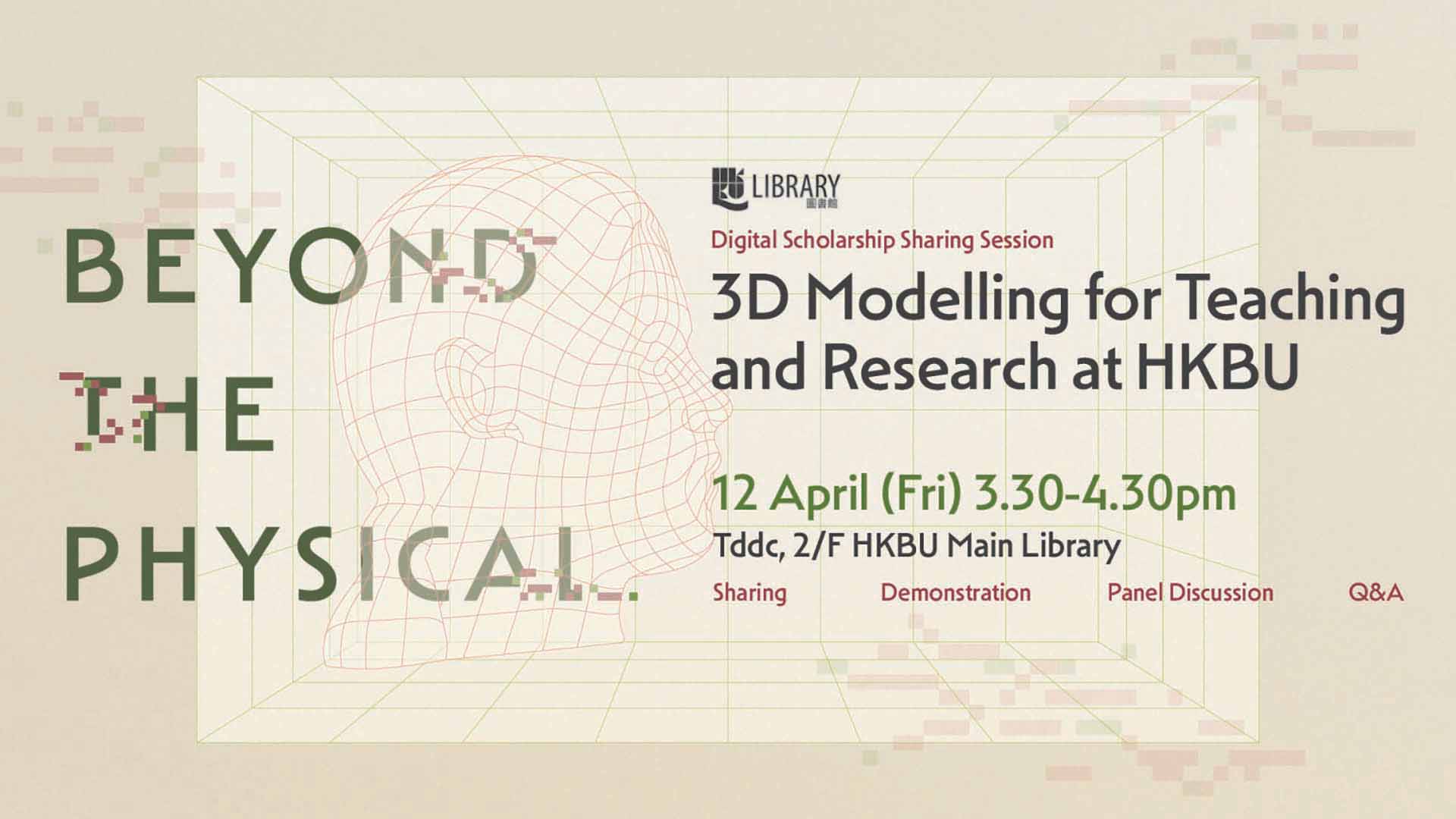
Beyond the Physical - 3D Modelling for Teaching and Research at HKBU
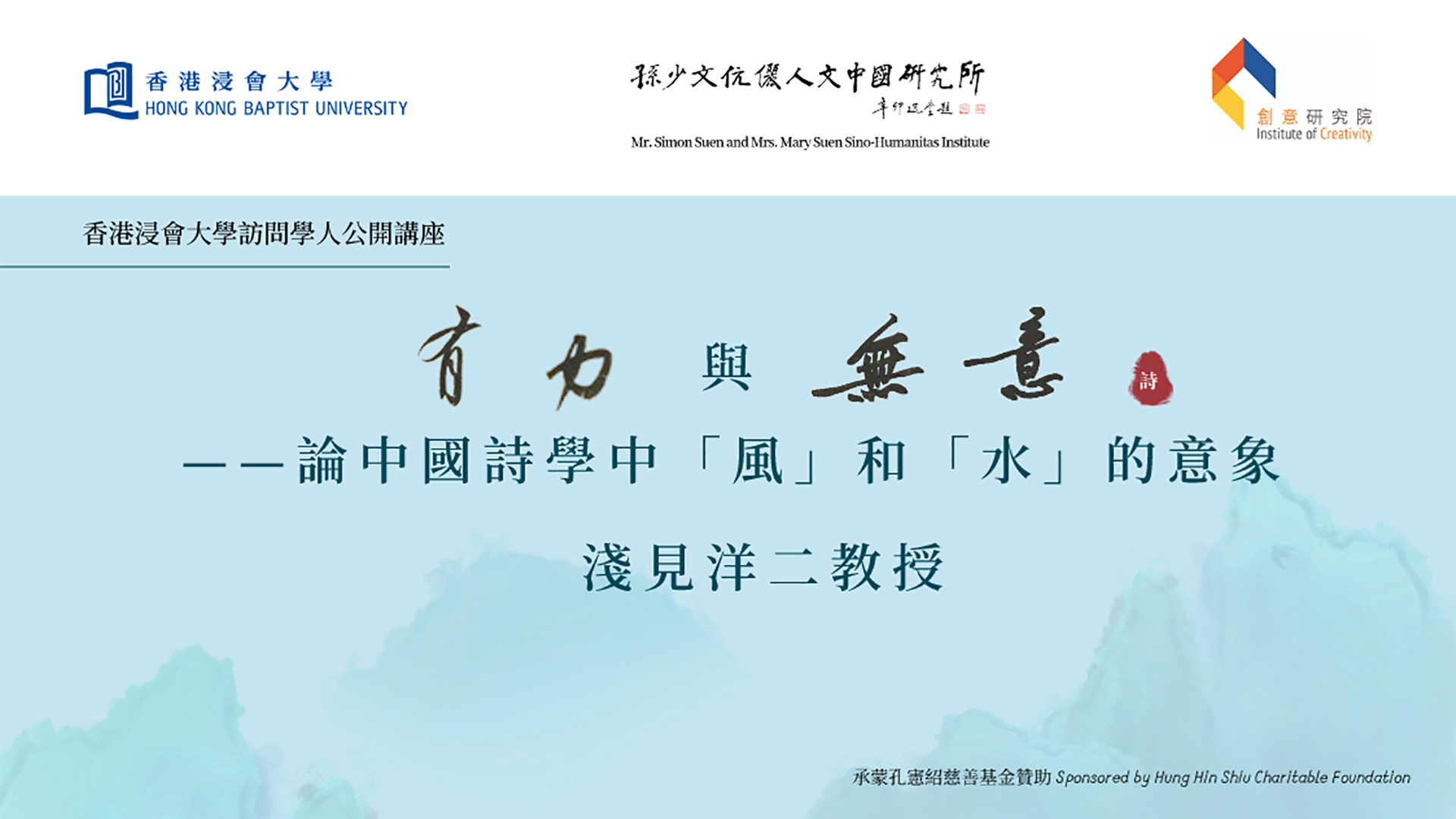
「有力」与「无意」——论中国诗学中「风」和「水」的意象
Teaching Videos 教學影片
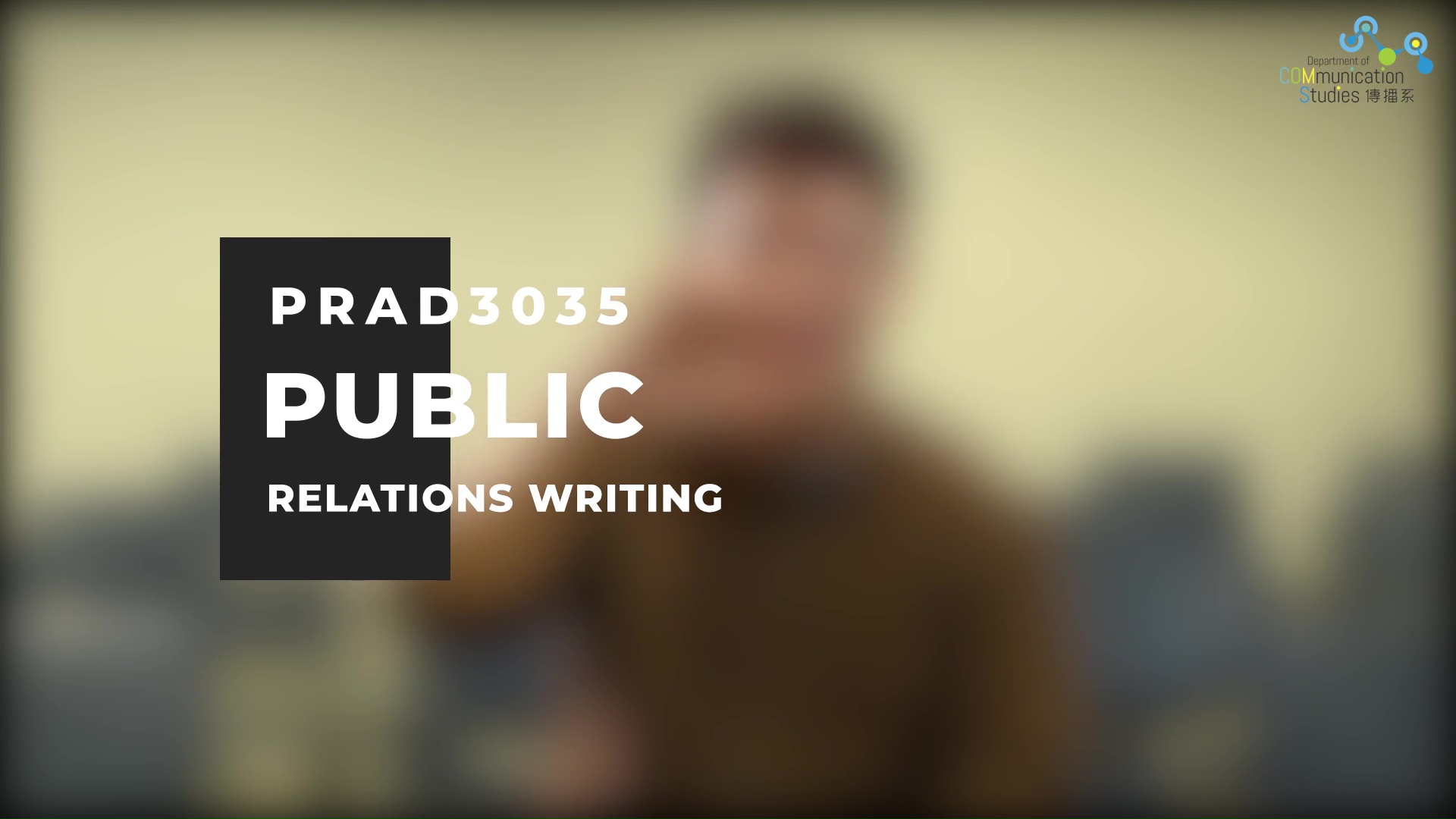
PRAD3035 Public Relations Writing
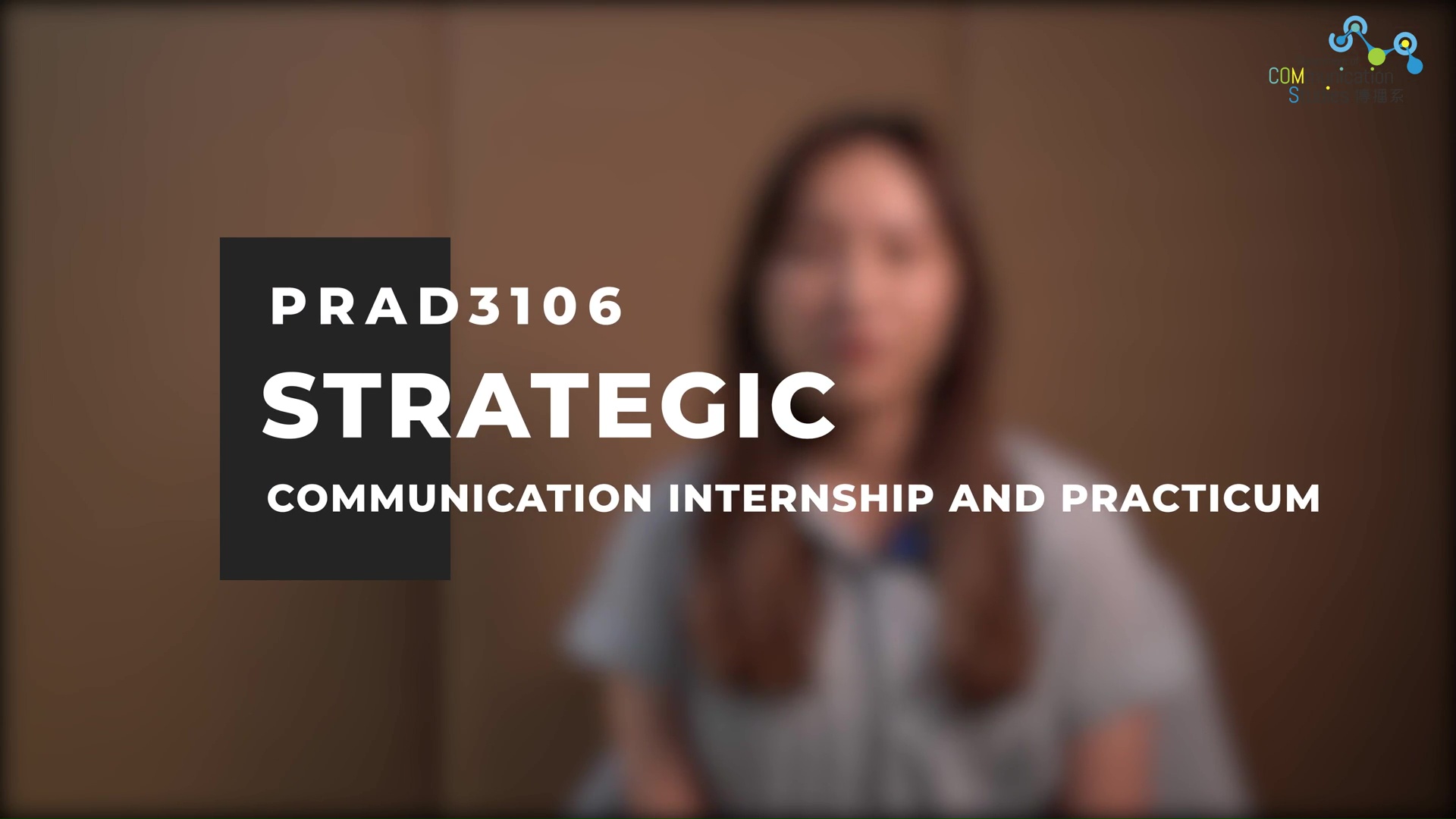
PRAD3106 Strategic Communication Internship and Practicum
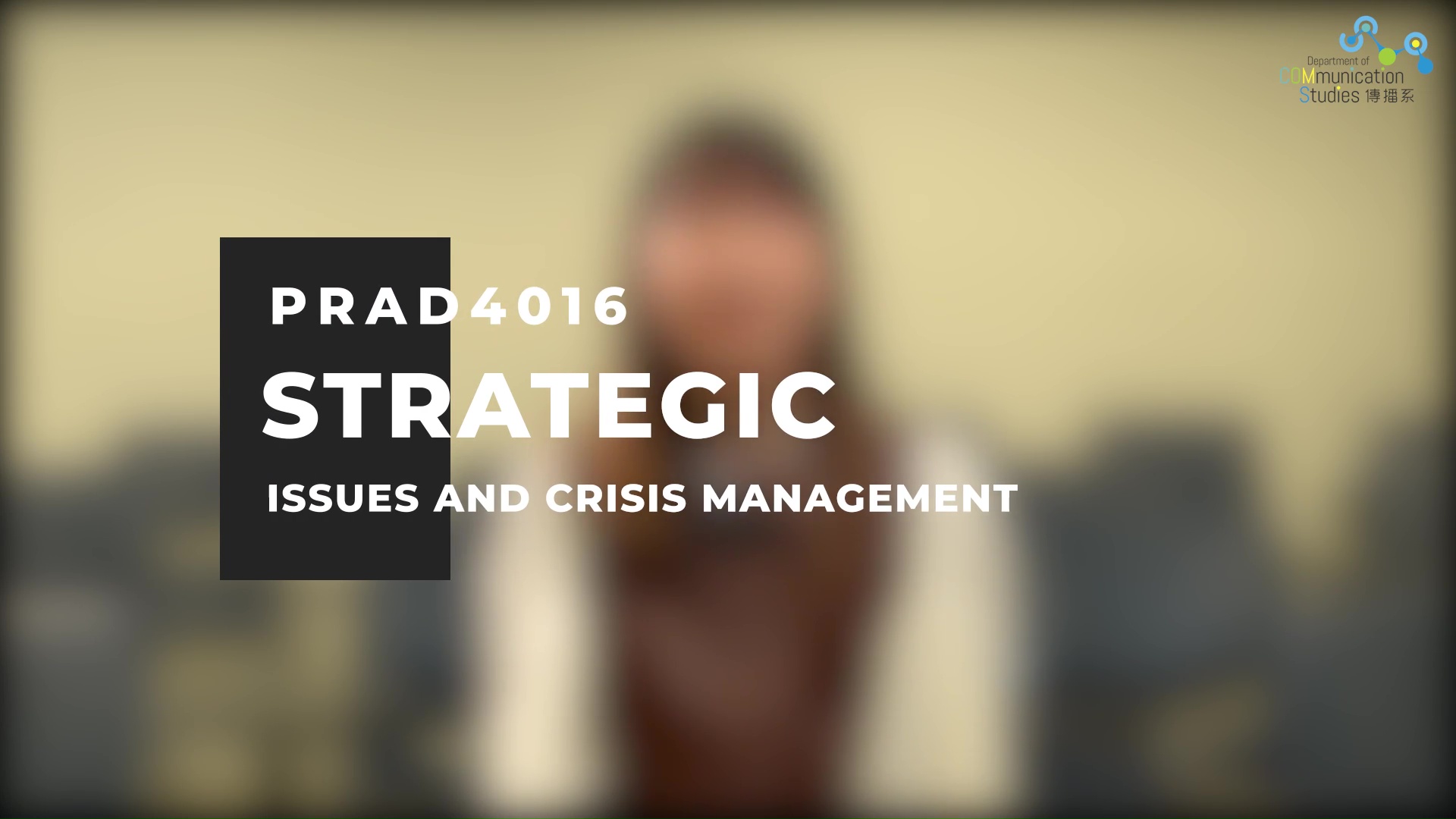
PRAD4016 Strategic Issues and Crisis Management
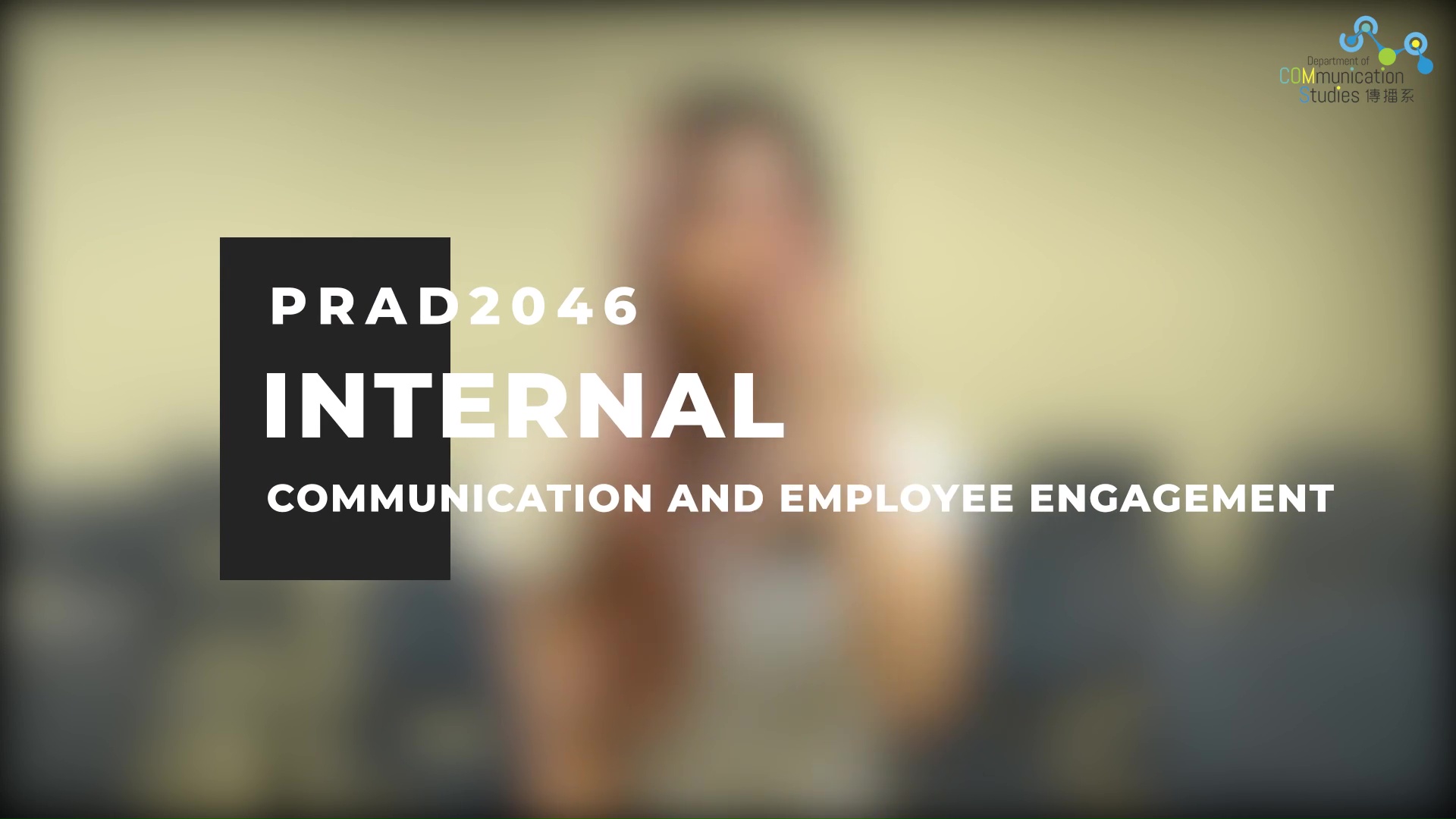
PRAD2046 Internal Communication and Employee Engagement
Digital Scholarship Grant Projects 數碼研究項目
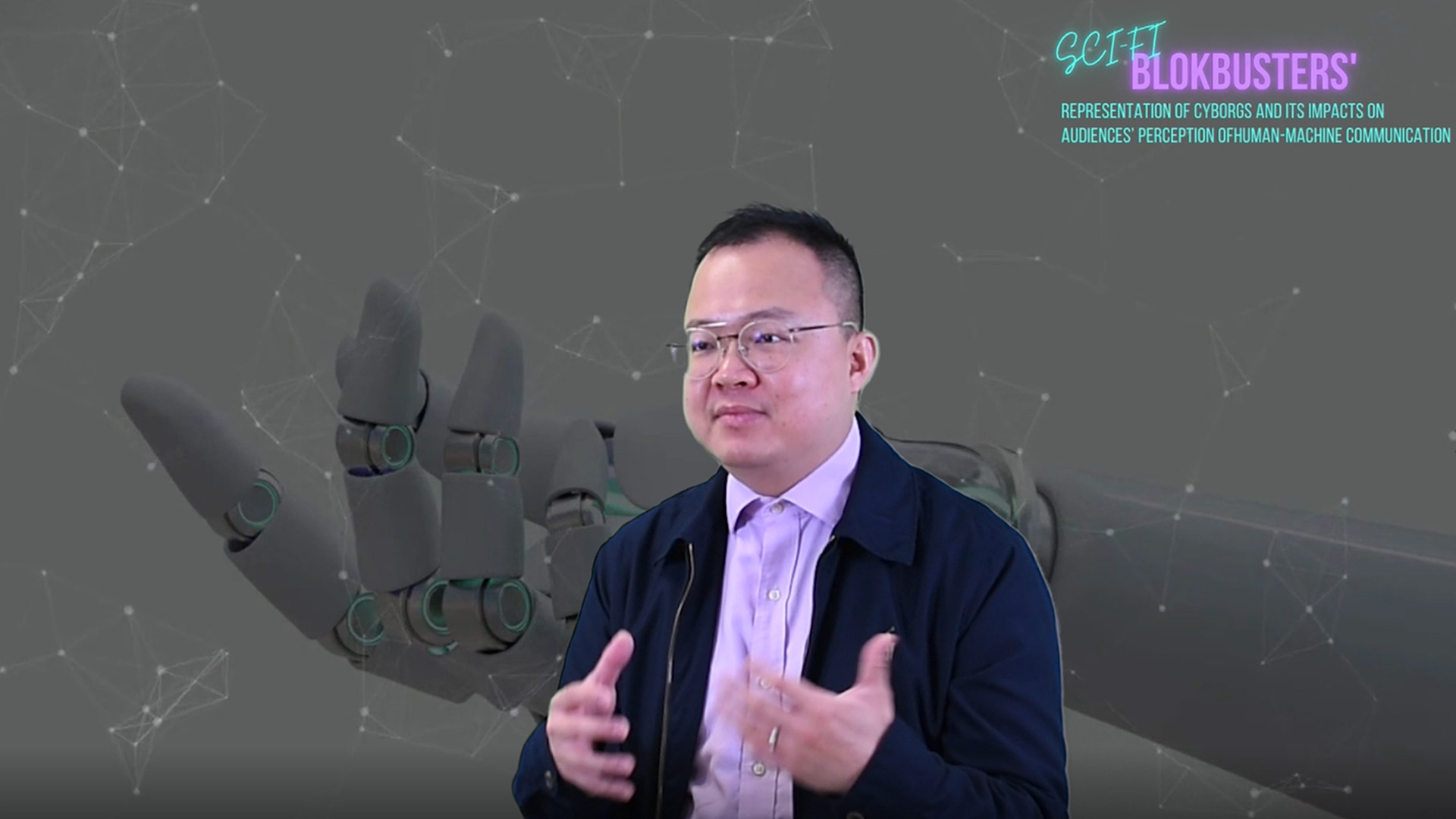
Digital Scholarship Grant Projects - Sci-fi Blockbusters' Representation of Cyborgs and its impacts on Audiences' Perception of Human-machine Communication
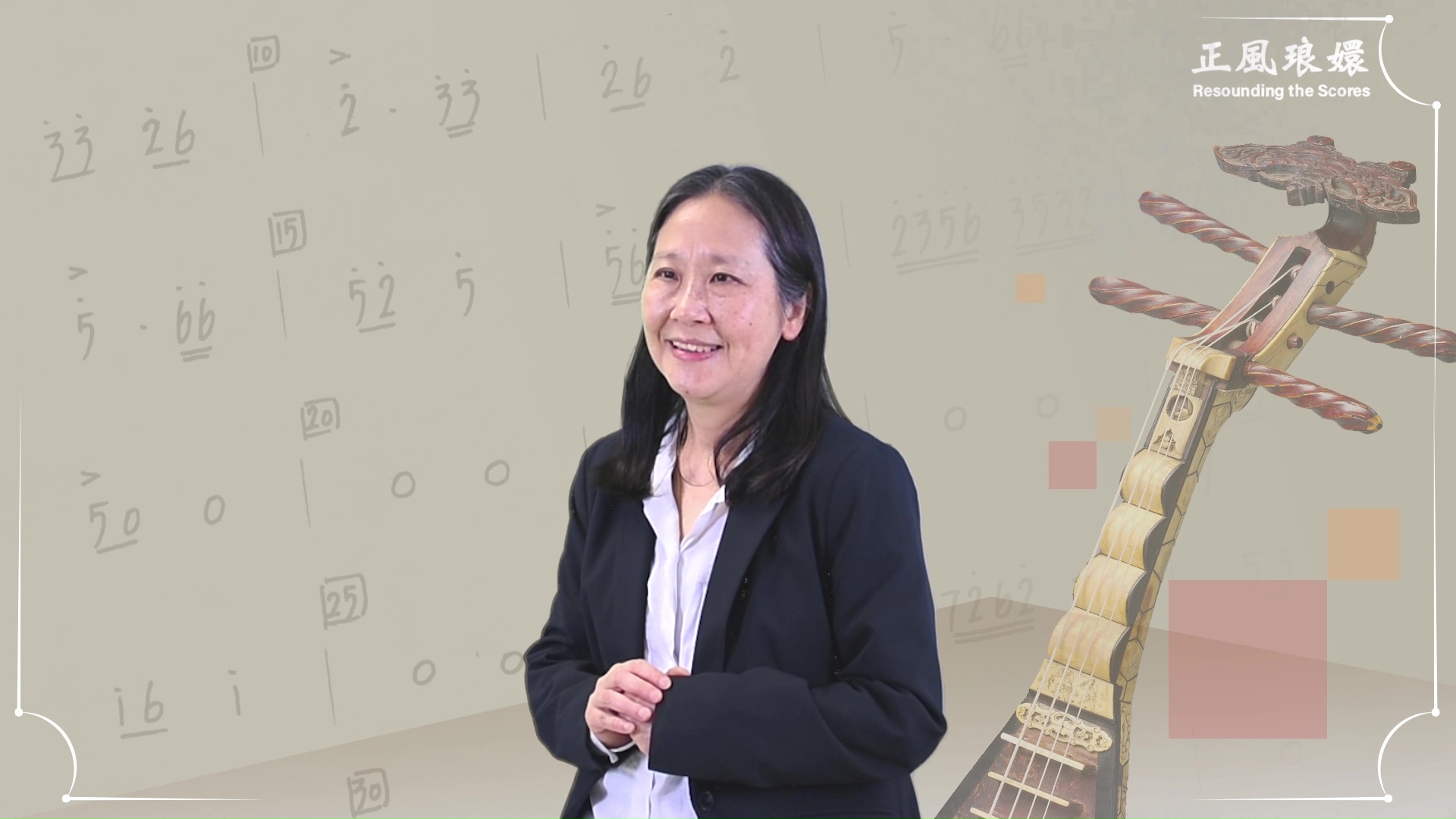
Digital Scholarship Grant Projects - 正風琅嬛 Resounding the Scores
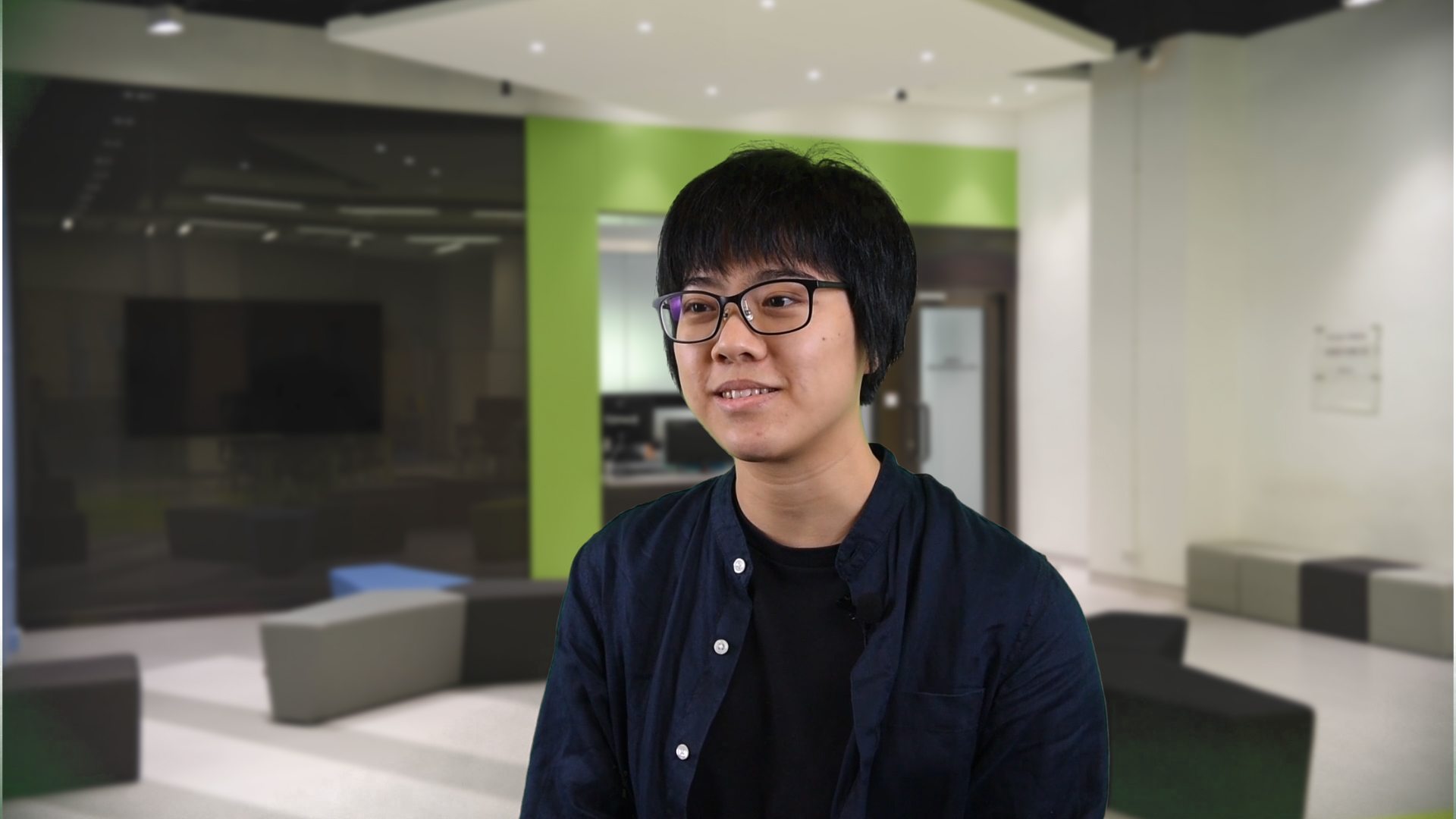
Digital Scholarship Grant Projects - Hong Kong Facebook News Analytics Dashboard
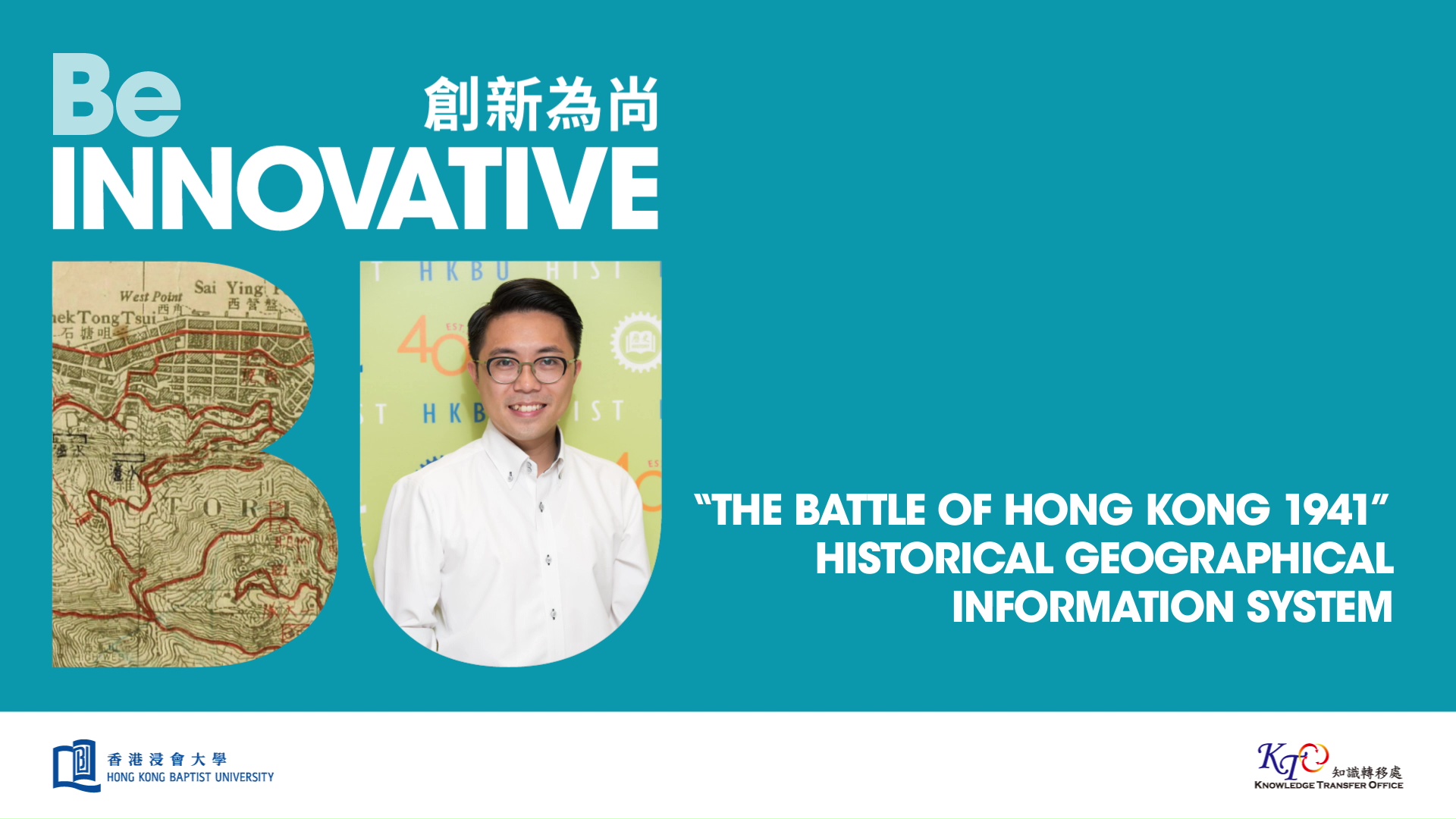
Digital Scholarship Grant Projects - The Battle of Hong Kong 1941: A Spatial History Project
Other videos 其他影片
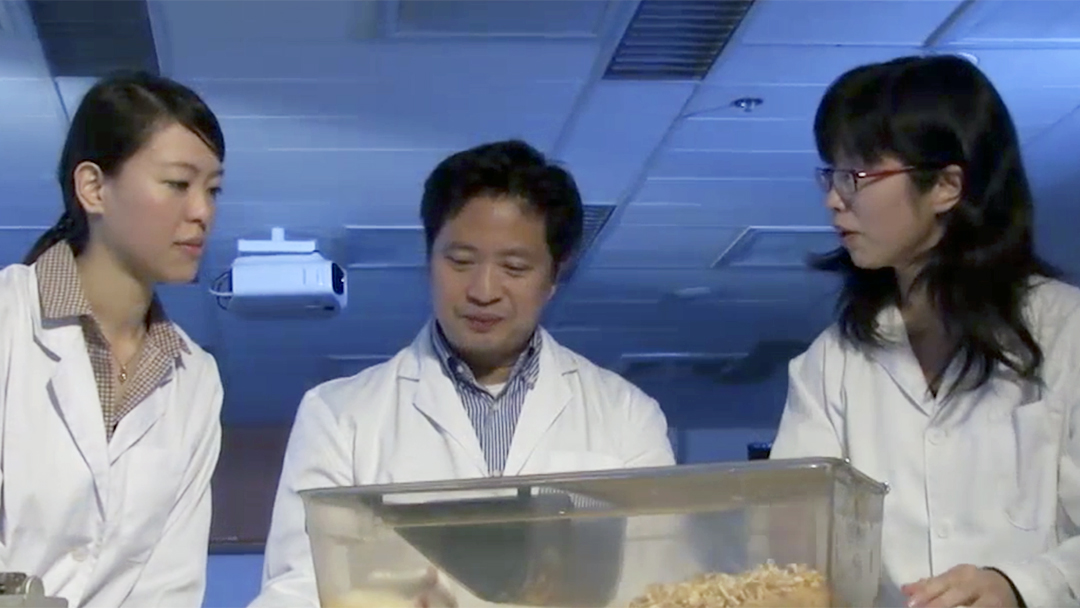
In Vivo Extraction of Neural Stem Cells in the Brain using Novel Nanomaterials
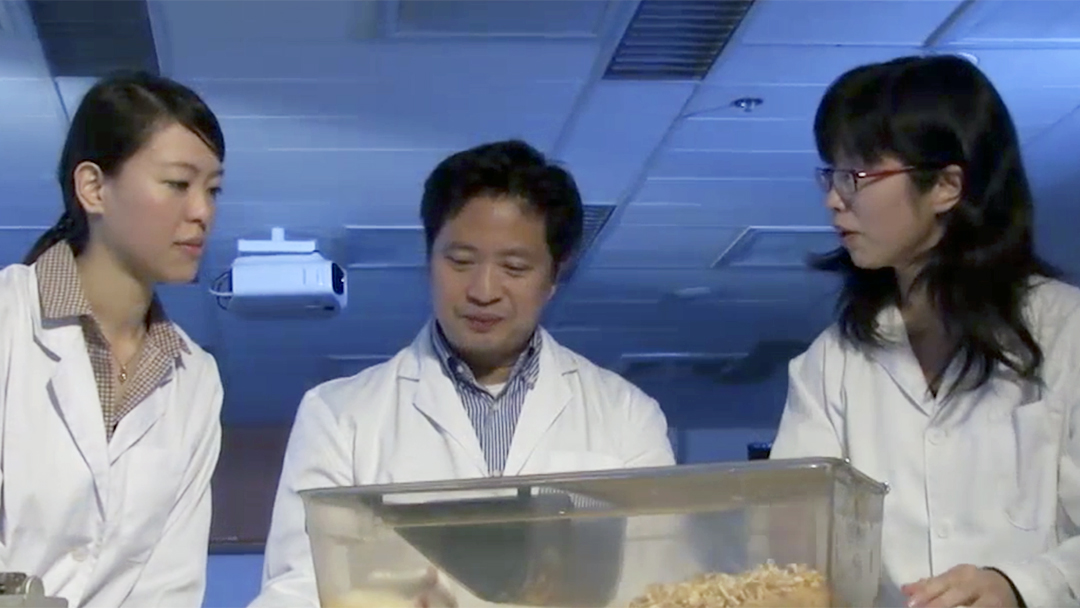
個性化的神經幹細胞用於治療腦退化疾病和腦損傷
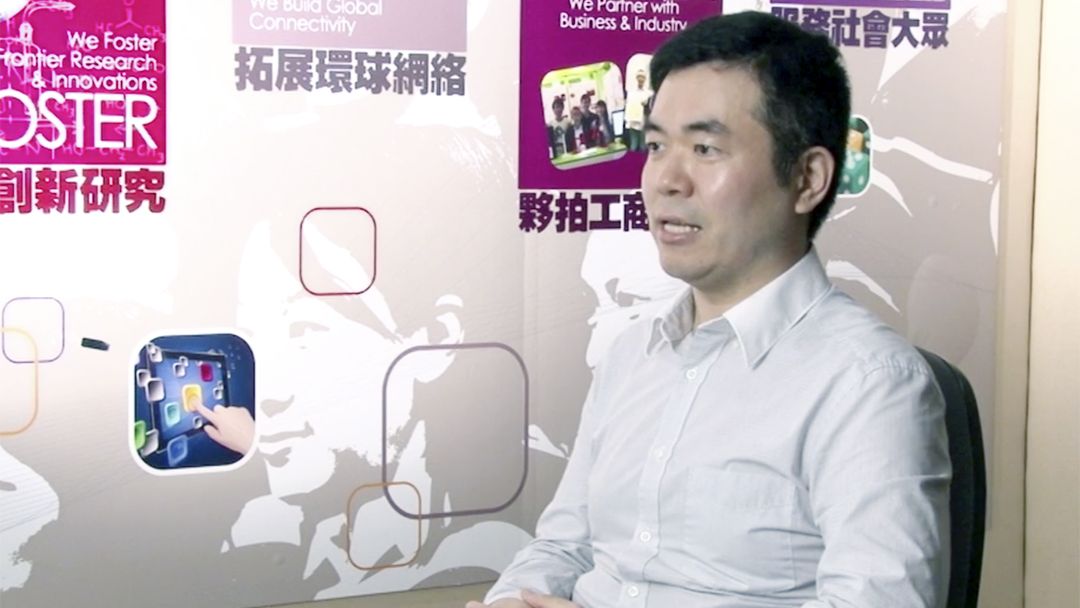
A Cloud-Computing Middleware for Providing Proximity Information to Mobile Geo-Social Networks
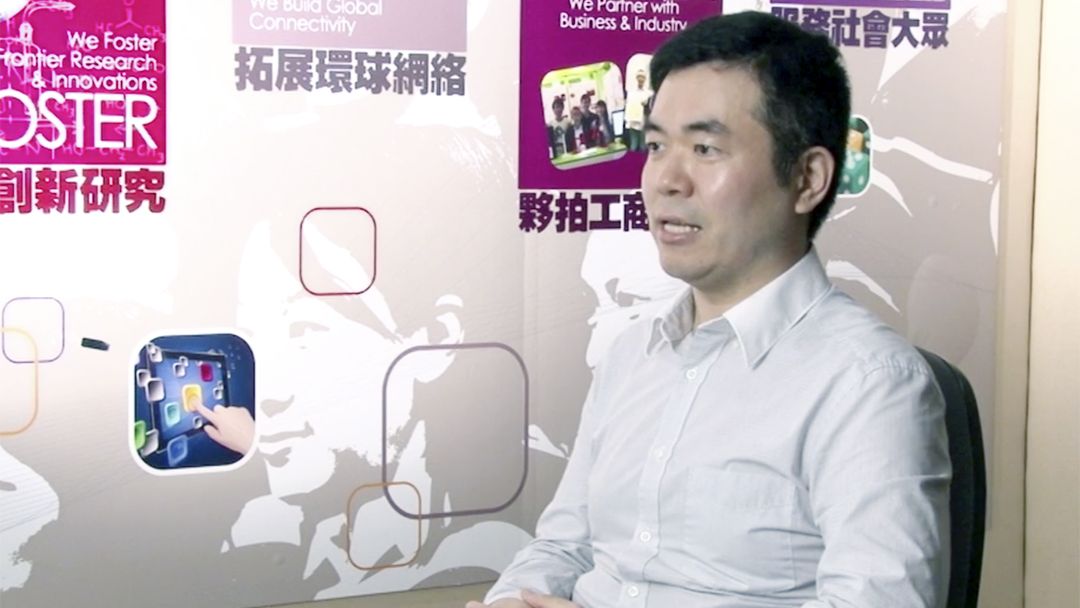